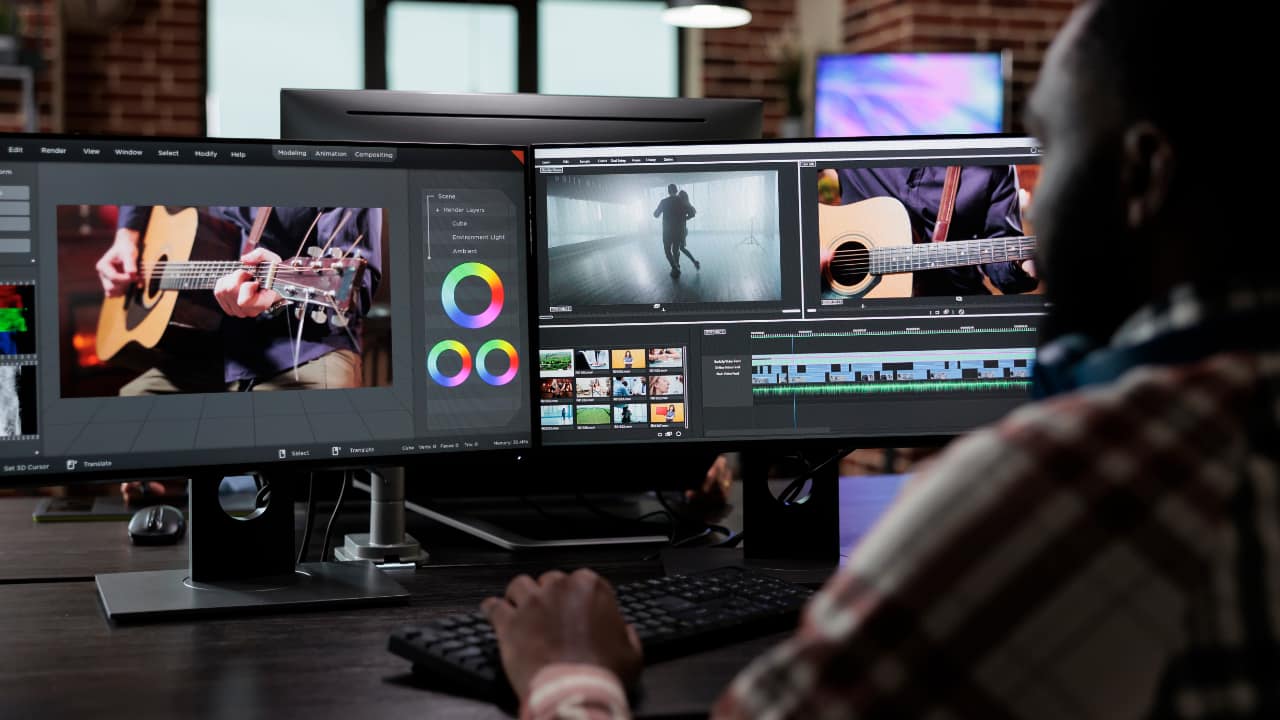
AI occupies a domain that is neither obviously analogue nor digital. Can it ever be the basis for a professional professional video recording - or even a post-production workflow?
For me, the analogue vs digital debate is over, with a simple, unarguable conclusion, which is that if you have sufficiently high-resolution sampling (and that includes both bit depth and sample rate), then you have near-perfect reproduction. One quick qualification: you have near-perfect reproduction of what entered the Analogue to Digital converter, not necessarily of reality, but that’s a debate about microphones and sensors.
In other words, there’s nothing intrinsic about digitisation that should distort or, in any other way, change the original signal.
To anyone not familiar with the sampling process, that may sound surprising. And analogue diehards probably won’t be satisfied with the mathematical reality that if you sample deep and high enough, you’re not missing anything useful from the original signal.
None of this is to say that I don’t like vinyl records, reel-to-reel tape or even 8mm cine film. It’s just that these are far more of an approximation of reality than a good digital recording, with often a healthy dose of character. There is something intrinsically warm and engaging about analogue recordings, nearly every nuance of which can now be simulated digitally.
The link between generative and analogue
I could write for days on this topic and enjoy mulling it over with dissenters from my own views. But today, the meaning of analogue is about to be stretched further than we previously thought was conceptually possible. Because I’m going to argue that generative AI is in some ways analogous to an analogue process. Or is it?
Everything about the phrase “Generative Artificial Intelligence” suggests that it’s not going to be faithful to the original. But that doesn’t have to be the case. What about noise removal? Noise can mask details that weren’t visible in the original picture, and AI can put them back. Or, at least, appear to put them back. Whether or not this is acceptable depends on the context. If the picture is a wedding portrait, and some details of the Bride’s dress aren’t as sharp as they should be, few would object to AI redressing (literally) the situation. But if the image was crucial evidence in a case around passport forgery, it would be likely to be thrown out of court, as it would essentially represent tampering with the evidence.
It’s easy to be distracted by the fact that AI runs on digital processors. These create neural networks that behave anything but digitally. It’s this relationship that is like that of digital sampling of analogue phenomena. In the end, the samples are not relevant to the output (other than as a means to reproducing it). When you digitally record an analogue musical instrument, the end result that you hear is an analogue instrument.
And so it is with AI. When you ask generative AI to create a scene, it doesn’t do it with pixels; it does it with concepts. If you ask it to draw a giraffe, it is that long-necked animal that is the output, and the current necessity of representing it as pixels (because that’s how our displays work) is not relevant. If, for some reason, our pixels were triangular or crescent-shaped, the AI would still work the same way. By the time you see the finished image, the pixels - and the digital processing - are irrelevant.
Improving reality?
What is the point of all this? It is, perhaps, that we will be able to use AI not only to “generate” something that never existed but also to “generate” reality. Show it the output from a camera’s sensor, and you can ask it to generate that same image, but better. Perhaps with more resolution, more detail, and of course, subtly modified to fit another aspect ratio or with a different colour grade.
This process essentially describes an AI codec. Instead of using mathematics like discrete cosine transformations to compress the image with as little visual loss as possible, you would task a specialist AI model with representing a scene in a conceptual domain. Depending on how this is done, it might even take more data than the original, but it seems likely that part of the model could use its own “intelligence” to look at how to reduce the amount of data needed to reproduce the same image.
And as we now know from experimenting with generative models, there are almost no limits. Post-production would become a matter of prompt engineering. And AI would also take part in optimising those prompts. So, yes, you could ask the model to “make this scene look like it was shot on a planet with two suns and a methane atmosphere”, but you could also use conventional colour grading UIs to control the AI process as well.
This won’t happen overnight. Output quality matters, and getting it right will be tricky. Ultimately, what we’re describing here is a semantic layer in a post-production technology workflow. It’s where you tell the workflow what you want the result to be. How we get to this stage is perhaps indistinct from today’s perspective, but there are stages we can build to get there. It’s what I call a “cognitive workflow”. It’s extremely speculative at the moment, but in a future article, I will describe the stages we need to build a workflow that is fully optimised for AI.
Tags: Post & VFX AI
Comments